Intelligent Design
"Swarm" Science: Why the Myth of Artificial Intelligence Threatens Scientific Discovery
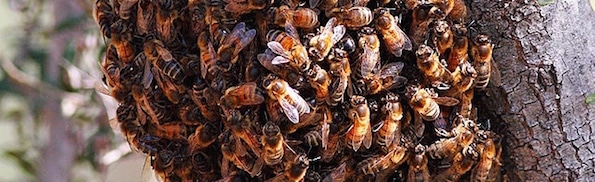
In the last year, two major well-funded efforts have launched in Europe and in the U.S. aimed at understanding the human brain using powerful and novel computational methods: advanced supercomputing platforms, analyzing peta- and even exabyte datasets, using machine learning methods like convolutional neural networks (CNNs), or “Deep Learning.”
At the Swiss Federal Institute of Technology in Lausanne (EPFL), for instance, the Human Brain Project is now underway, a ten-year effort funded by the European Commission to construct a complete computer simulation of the human brain. In the U.S., the Obama Administration has provided an initial $100 million in funding for the newly launched Brain Research Through Advanced Neurotechnologies (BRAIN) Initiative, with funding projected to reach $3 billion in the next ten years. Both projects are billed as major leaps forward in our quest to gain a deeper understanding of the brain — one of the last frontiers of scientific discovery.
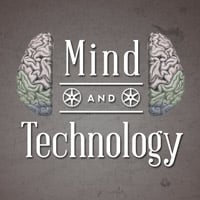
Predictably, given today’s intellectual climate, both projects are premised on major confusions and fictions about the role of science and the powers of technology.
The myth of evolving Artificial Intelligence, for one, lies at the center of these confusions. While the U.S. BRAIN Initiative is committed more to the development of measurement technologies aimed at mapping the so-called human connectome — the wiring diagram of the brain viewed as an intricate network of neurons and neuron circuits — the Human Brain Project more explicitly seeks to engineer an actual, working simulation of a human brain.
The AI myth drives the HBP vision explicitly, then, even as ideas about Artificial Intelligence and the powers of data-driven methods (aka “Big Data”) undergird both projects. The issues raised today in neuroscience are large, significant, and profoundly troubling for science. In what follows, I’ll discuss Artificial Intelligence and its role in science today, focusing on how it plays out so unfortunately in neuroscience, and in particular in the high-visibility Human Brain Project in Switzerland.
AI and Science
AI is the idea that computers are becoming intelligent in the same sense as humans, and eventually to even a greater degree. The idea is typically cast by AI enthusiasts and technologists as forward-thinking and visionary, but in fact it has profoundly negative affects on certain very central and important features of our culture and intellectual climate. Its eventual effects are to distract us from using our own minds.
The connection here is obvious, once you see it. If we believe that the burden of human thinking (and here I mean, particularly, explaining the world around us) will be lessened because machines are rapidly gaining intelligence, the consequence to science if this view is fictitious can only be to diminish and ultimately to imperil it.
At the very least, we should expect scientific discovery not to accelerate, but to remain in a confused and stagnant state with this set of ideas. These ideas dominate today.
Look at the history of science. Scientists have grand visions and believe they can explain the world by contact of the rational mind with nature. One thinks of Einstein, but many others as well: Copernicus, Galileo, Newton, Maxwell, Hamilton, Heisenberg, even Watson and Crick.
Copernicus, for instance, became convinced that the entire Ptolemaic model of the solar system stemmed from false theory. His heliocentric model is a case study in the triumph of the human mind not to analyze data but effectively to ignore it — seeking a more fundamental explanation of observation in a rational vision that is not data-driven but prior to and more fundamental than what we collect and view (the “data”). Were computers around back then, one feels that Copernicus would have ignored their results too, so long as they were directed at analyzing geocentric models. Scientific insight here is key, yesterday and today.
Yet the current worldview is committed, incessantly and obsessively, to reducing scientific insight to “swarms” of scientists working on problems, by each making little contributions to a framework that is already in place. The Human Brain Project here is paradigmatic: the “swarm” language is directly from a key HBP contributor Sean Hill (in the recent compilation The Future of the Brain edited by Gary Marcus, whom I like).
The swarm metaphor evokes thoughts of insects buzzing around, fulfilling pre-ordained roles. So if we’re convinced that in a Human-Technology System the “technology” is actually becoming humanly intelligent (the AI myth), the set of social and cultural beliefs begin to change to accommodate a technology-centered worldview. This, however, provides very little impetus for discovery.
To the extent that individual minds aren’t central to the technology-driven model of science, then “progress” based on “swarm science” further reinforces the belief that computers are increasingly responsible for advances. It’s a self-fulfilling vision; the only problem is that fundamental insights, not being the focus anyway, are also the casualties of this view. If we’re living in a geocentric universe with respect to, say, neuroscience still, the model of “swarm” science and data-driven analysis from AI algorithms isn’t going to correct us. That’s up to us: in the history of science, today, and in our future.
An example. Neuroscientists are collecting massive datasets from neural imaging technologies (not itself a bad thing), believing that machine-learning algorithms will find interesting patterns in the data. When the problem is well defined, this makes sense.
But reading the literature, it’s clear that the more starry-eyed among the neuroscientists (like Human Brain Project director Henry Markram) also think that such an approach will obviate the need for individual theory in favor of a model where explanation “emerges” from a deluge of data.
This is not a good idea. For one thing, science doesn’t work that way. The “swarm-and-emerge” model of science would seem ridiculous were it not for the belief that such massive quantities of data run on such powerful computing resources (“massive” and “powerful” is part of the emotional component of this worldview) could somehow replace traditional means of discovery, where scientists propose hypotheses and design specific experiments to generate particular datasets to test those hypotheses.
Now, computation is supposed to replace all that human-centered scientific exploration — Markram himself has said publicly that the thousands of individual experiments are too much for humans to understand. It may be true that the volume of research is daunting, but the antidote can hardly be to force thousands of labs to input data into a set of APIs that presuppose a certain, particular theory of the brain! (This is essentially what the Human Brain Project does.) We don’t have the necessary insights, yet, in the first place.
Even more pernicious, the belief that technology is “evolving” and getting closer and closer to human intelligence gives less and less an impetus to people to fight for traditional scientific practice, centered on discovery. If human thought is not the focus anymore, why empower all those individual thinkers? Let them “swarm,” instead, around a problem that has been pre-defined.
This too is an example of how the AI myth also encourages a kind of non-egalitarian view of things, where a few people are actually telling everyone else what to do, even as the model is supposed to be communitarian in spirit. This gets us a little too far off topic presently, but is a fascinating case study in how false narratives are self-serving in subtle ways.
Back to science. In fact the single best worldview for scientific discovery is simple: human minds explain data with theory. Now, but only after we have this belief, we can and should insert: and our technology can help us. Computation is a tool — a very powerful one, but as it isn’t becoming intelligent in the sense of providing theory for us, we can’t then jettison our model of science, and begin downplaying or disregarding the theoretical insights that scientists (with minds) provide.
This is a terrible idea. It’s just terrible. It’s no wonder that any major scientific successes in the last decade have been largely engineering-based, like the Human Genome Project. No one has the patience, or even the faith, to fund smaller-scale and more discovery-based efforts.
The idea, once again, is that the computational resources will somehow replace traditional scientific practice, or “revolutionize it” — but as I’ve been at pains to argue, computation isn’t “smart” in the way people are, and so the entire AI Myth is not positive, or even neutral, but positively threatening to real progress.
The End of Theory? Maybe So
Hence when Chris Andersen wrote in 2007 that Big Data and super computing (and machine learning or i.e., induction) meant the “End of Theory,” he echoed the popular Silicon Valley worldview that machines are evolving a human — and eventually a superhuman — intelligence, and he simultaneously imperiled scientific discovery. Why? Because (a) machines aren’t gaining abductive inference powers, and so aren’t getting smart in the relevant manner to underwrite “end of theory” arguments, and (b) ignoring the necessity of scientists to use their minds to understand and explain “data” is essentially gutting the central driving force of scientific change.
To put this yet again on more practical footing, over five hundred neuroscientists petitioned the EU last year because a huge portion of funding for fundamental neuroscience research (over a billion euro) went to the Human Brain Project, which is an engineering effort that presupposes that fundamental pieces of theory about the brain are in place. The only way a reasonable person could believe that, is if he were convinced that the Big Data/AI model would yield those theoretical fruits somehow along the way. When pressed, however, the silence as to how exactly that will happen is deafening.
The answer Markram and others want to provide — if only sci-fi arguments worked on EU officials or practicing neuroscientists — is that the computers will keep getting “smarter.” And so that myth is really at the root of a lot of current confusion. Make no mistake, the dream of AI is one thing, but the belief that AI is around the corner and inevitable is just a fiction, and potentially a harmful one at that.
Image by Taken byfir0002 | flagstaffotos.com.auCanon 20D + Canon 400mm f/5.6 L (Own work) [GFDL 1.2], via Wikimedia Commons.