Evolution
Intelligent Design
Evolutionary Informatics: Marks, Dembski, and Ewert Demonstrate the Limits of Darwinism
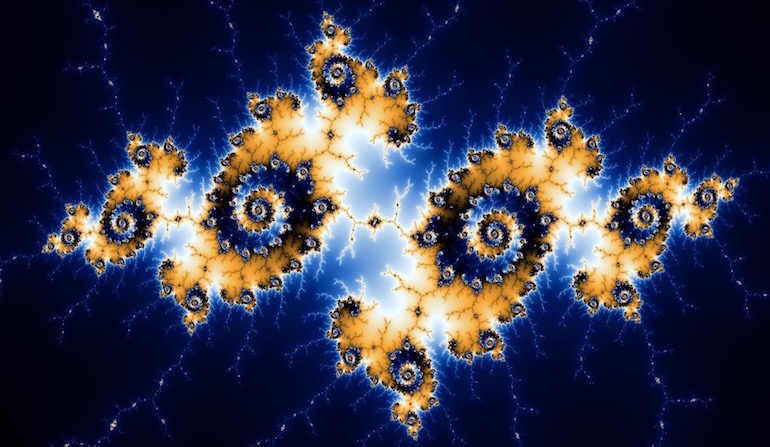
In the evolution debate, a key issue is the ability of natural selection to produce complex innovations. In a previous article, I explained based on engineering theories of innovation why the small-scale changes that drive microevolution should not be able to accumulate to generate the large-scale changes required for macroevolution. This observation perfectly corresponds to research in developmental biology and to the pattern of the fossil record. However, the limitations of Darwinian evolution have been demonstrated even more rigorously from the fields of evolutionary computation and mathematics. These theoretical challenges are detailed in a new book out this week, Introduction to Evolutionary Informatics.
Authors Robert Marks, William Dembski, and Winston Ewert bring decades of experience in search algorithms and information theory to analyzing the capacity of biological evolution to generate diverse forms of life. Their conclusion is that no evolutionary process is capable of yielding different outcomes (e.g., new body plans), being limited instead to a very narrow range of results (e.g., finches with different beak sizes). Rather, producing anything of significant complexity requires that knowledge of the outcomes be programmed into the search routines. Therefore, any claim for the unlimited capacity of unguided evolution to transform life is necessarily implausible.
The authors begin their discussion by providing some necessary background. They present an overview of how information is defined, and define the standard measures of KCS (Kolmogorov–Chaitin-Solomonov) complexity and Shannon information. The former provides that minimum number of bits required to repeat a pattern — the maximum compressibility. The latter relates to the log of the probability of some pattern emerging as an outcome. For instance, the probability of flipping five coins and having them all land on heads is 1/32. The information content of HHHHH is then the negative log (base 2) of 1/32, which is 5 bits. More simply, a specific outcome of 5 coin flips is equivalent to 5 bits of information.
They describe how searches in engineering for some design outcome involve the three components of domain expertise, design criteria, and iterative search. The process involves creating a prototype and then checking to see if it meets the criteria, which functions as a teleological goal. If the initial design does not, the prototype is refined and the test repeated. The greater the domain expertise, the more efficiently adjustments are made, so fewer possibilities need to be tested. Success can then be achieved more quickly.
They demonstrate this process with a homely example: cooking pancakes. The first case involves adjusting the times the pancakes were cooked on the front and on the backside. An initial pancake was cooked for two random times, and it was then tasted. Based on the taste, the times were then adjusted for the second iteration. This process was repeated until a pancake’s taste met some quality threshold. For future cases, additional variables were added, such as the amount of milk used in the batter, the temperature setting, and the added amount of salt. If each variable were assigned a value between 1 and 10, such as the ten settings on the stove burner, the number of possible trials increased by a factor of 10 for each new variable. The number of possibilities grows very quickly.
For several variables, if the taster had no knowledge of cooking, the time required to find a suitable outcome would likely be prohibitively long. However, with greater knowledge, better choices could be made to reduce the number of required searches. For instance, an experienced cook (that is, a cook with greater domain expertise) would know that the time on both sides should be roughly the same, and pancakes that are too watery require additional flour.
This example follows the basic approach to common evolutionary design searches. The main difference is that multiple trials can often be simulated on a computer at once. Then, each individual can be independently tested and altered. The components of each cycle include a fitness function (aka oracle) to define that status of an individual (e.g., taste of the pancake), a method of determining which individuals are removed and which remain or are duplicated, and how individuals are altered for the next iteration (e.g., more milk). The authors provide several examples of how such evolutionary algorithms could be applied to different problems. One of the most interesting examples they give is how NASA used an evolutionary algorithm to bend a length of wire into an effective X-band antenna.
In this way, the authors demonstrate the limitations of evolutionary algorithms. The general challenge is that all evolutionary algorithms are limited to converging on a very narrow range of results, a boundary known as Basener’s Ceiling. For instance, a program designed to produce an antenna will at best converge to the solution of an optimal antenna and then remain stuck. It could never generate some completely different result, such as a mousetrap. Alternatively, an algorithm designed to generate a strategy for playing checkers could never generate a strategy for playing backgammon. To change outcomes, the program would have to be deliberately adjusted to achieve a separate predetermined goal. In the context of evolution, no unguided process could converge on one organism, such as a fish, and then later converge on an amphibian.
This principle has been demonstrated both in simulations and in experiments. The program Tierra was created in the hope of simulating large-scale biological evolution. Its results were disappointing. Several simulated organisms emerged, but their variability soon hit Basener’s Ceiling. No true novelty was ever generated but simply limited rearrangements of the initially supplied information. We have seen a similar result in experiments on bacteria by Michigan State biologist Richard Lenski. He tracked the development of 58,000 generations of E. coli. He saw no true innovation but primarily the breaking of nonessential genes to save energy, and the rearrangement of genetic information to access pre-existing capacities, such as the metabolism of citrate, under different environmental stresses. Changes were always narrow in scope and limited in magnitude.
The authors present an even more defining limitation, based on the No Free Lunch Theorems, which is known as the Conservation of Information (COI). Stated simply, no search strategy can on average find a target more quickly than a random search unless some information about that target is incorporated into the search process. As an illustration, imagine someone asking you to guess the name of a famous person, but without giving you any information about that individual. You could use many different guessing strategies, such as listing famous people you know in alphabetical order, or by height, or by date of birth. No strategy could be determined in advance to be better than a random search.
However, if you were allowed to ask a series of questions, the answers would give you information that could help limit or guide your search. For instance, if you were told that the famous person was contemporary, that would dramatically reduce your search space. If you then learned the person was an actor, you would have even more guidance on how to guess. Or you might know that the chooser is a fan of science fiction, in which case you could focus your guessing on people associated with the sci-fi genre.
We can understand the theorem more quantitatively. The size of your initial search space could be defined in terms of the Shannon Information measure. If you knew that one of 32 famous people was the target, the search space would correspond to log (base 2) of 32, which is 5 bits. This value is known as the endogenous information of the search. The information given beforehand to assist the search is known as the active information. If you were given information that eliminated all but 1/4 of the possible choices, you would have log (base 2) of 4, which is 2 bits of active information. The information associated with finding the target in the reduced search space is then log (base 2) of 32/4, which is 3 bits. The search-related information is conserved: 5 bits (endogenous) = 2 bits (active) + 3 bits (remaining search space).
The COI theorem holds for all evolutionary searches. The NASA antenna program only works because it uses a search method that incorporates information about effective antennas. Other programs designed to simulate evolution, such as Avida, are also provided with the needed active information to generate the desired results. In contrast, biological evolution is directed by blind natural selection, which has no active information to assist in searching for new targets. The process is not helped by changes in the environment or coevolutionary interactions, which alter the fitness landscape, since such changes contain no active information related to a radically different outcome.
In the end, the endogenous information associated with finding a new body plan or some other significant modification is vastly greater than that associated with the search space that biological offspring could possibly explore in the entire age of the universe. Therefore, as these authors forcefully show, in line with much previous research in the field of intelligent design, all radical innovations in nature required information from some outside intelligent source.
Image: Mandelbrot set, detail, by Binette228 (Own work) [CC BY-SA 3.0], via Wikimedia Commons.