Intelligent Design
Medicine
Physics, Earth & Space
Mendel’s Peas and More: Inferring Data Falsification in Science
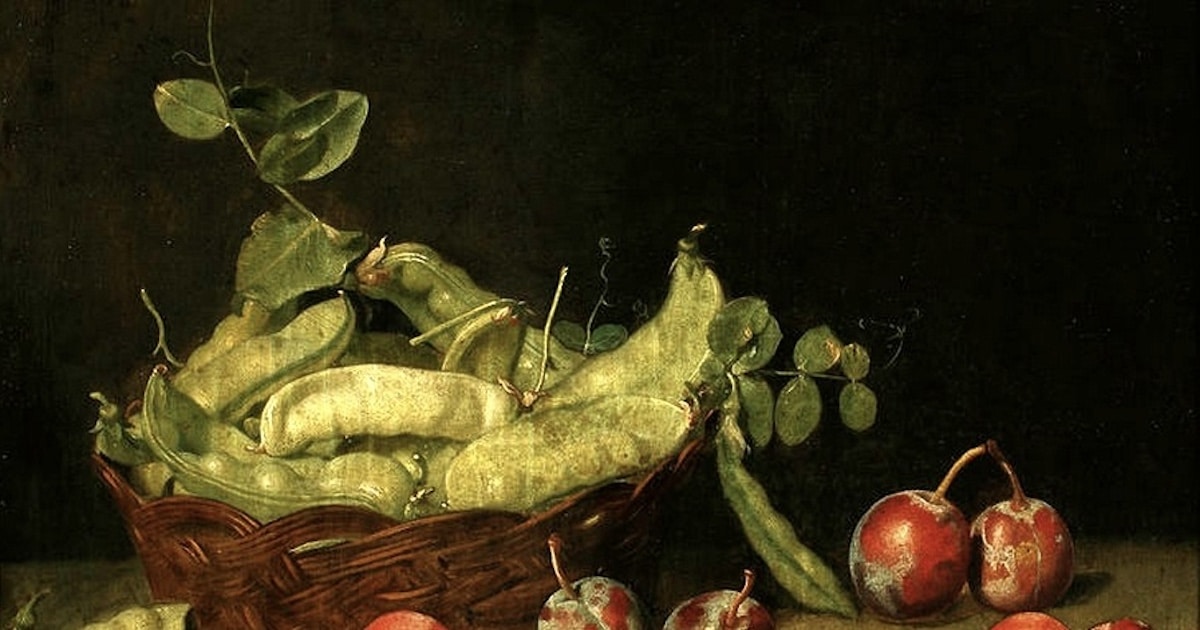
Editor’s note: We are delighted to welcome the new and greatly expanded second edition of The Design Inference, by William Dembski and Winston Ewert. The following is excerpted from Chapter 2, “A Sampler of Design Inferences.”
Drawing design inferences is not an obscure or rare occurrence — it happens daily. We distinguish between a neatly folded pile of clothes and a random heap, between accidental physical contact and a deliberate nudge, between doodling and a work of art. Furthermore, we make important decisions based on this distinction. This chapter examines a variety of areas where we apply the design inference. In each case, what triggers a design inference is a specified event of small probability.
The eminent statistician Ronald Aylmer Fisher uncovered a classic case of data falsification when he analyzed Gregor Mendel’s data on peas. Fisher inferred that “Mendel’s data were fudged,” as one statistics text puts it, because the data matched Mendel’s theory too closely. Interestingly, the coincidence that elicited this charge of data falsification was a specified event whose probability was roughly 4 in 100,000, or 1 in 25,000. By everyday standards, this probability will seem small enough, but it is huge compared to many of the probabilities we will be encountering. In any case, Fisher saw this probability as small enough to draw a design inference, concluding that Mendel’s experiment was compromised and charging Mendel’s gardening assistant with deception.
Slutsky — Fast and Furious
For a more recent example of data falsification in science, consider the case of UCSD heart researcher Robert A. Slutsky. Slutsky was publishing fast and furiously. At his peak, he was publishing one new paper every ten days. Intent on increasing the number of publications in his curriculum vitae, he decided to lift a two-by-two table of summary statistics from one of his articles and insert it — unchanged — into another article. Data falsification was clearly implicated because of the vast improbability that data from two separate experiments should produce the same summary table of statistics. When forced to face a review board, Slutsky resigned his academic position rather than try to explain how this coincidence could have occurred without any fault on his part. The incriminating two-by-two table that appeared in both articles consisted of four blocks each containing a three-digit number. Given therefore a total of twelve digits in these blocks, the odds would have been roughly 1 in a trillion (= 1012) that this same table might have appeared by chance twice in his research.
Why did Slutsky resign rather than defend a 1 in 1012 improbability? Why not simply attribute the coincidence to chance? There were three reasons. First, at the review board Slutsky would have had to produce the experimental protocols for the two experiments that supposedly gave rise to the identical two-by-two tables. If he was guilty of data falsification, these protocols would have incriminated him. Second, even if the protocols were lost, the sheer improbability of producing so unlikely a match between the two papers would have been enough to impugn the researcher’s honesty. Once a specification is in place (the two-by-two table in one paper here specifying the table in the other) and the probabilities become too small, the burden of proof, at least within the scientific community, shifts to the experimenter suspected of data falsification. In lay terms, Slutsky was self-plagiarizing. And third, Slutsky knew that this case of fraud was merely the tip of the iceberg. He had been committing other acts of research fraud right along, and these were now destined all to come into the open.
Moving from Medicine to Physics
Now consider the case of Jan Hendrik Schön, which parallels the Slutsky case almost point for point. On May 23, 2002, the New York Times reported on the work of “J. Hendrik Schön, 31, a Bell Labs physicist in Murray Hill, NJ, who has produced an extraordinary body of work in the last two and a half years, including seven articles each in Science and Nature, two of the most prestigious journals.” Despite this track record, his career was on the line. The New York Times reported further that Schön published “graphs that were nearly identical even though they appeared in different scientific papers and represented data from different devices. In some graphs, even the tiny squiggles that should arise from purely random fluctuations matched exactly.” (The identical graphs that did in Schön parallel the identical two-by-two tables that did in Slutsky.) Bell Labs therefore appointed an independent panel to determine whether Schön was guilty of “improperly manipulating data in research papers published in prestigious scientific journals.” The hammer fell in September 2002 when the panel concluded that Schön had indeed falsified his data, whereupon Bell Labs fired him.17
Exactly how a design inference was drawn in the Schön case is illuminating. In determining whether Schön’s numbers were made up fraudulently, the panel noted, if only tacitly, that the first published graph provided a pattern independently identified of the second and thus constituted the type of pattern that, in the presence of improbability, could negate chance to underwrite design (i.e., the pattern was a specification). And indeed, the match between the two graphs in Schön’s articles was highly improbable assuming the graphs arose from random processes (which is how they would have had to arise if, as Schön claimed, they resulted from independent experiments). As with the matching two-by-two tables in the Slutsky example, the match between the two graphs of supposedly random fluctuations would have been too improbable to occur by chance. With specification and improbability both evident, a design inference followed.
But, as noted earlier, a design inference, by itself, does not implicate any particular intelligence. So how do we know that Schön was guilty? A design inference shows that Schön’s data were cooked. It cannot, without further evidence, show that Schön was the chef. To do that required a more detailed causal analysis — an analysis performed by Bell Labs’ independent panel. From that analysis, the panel concluded that Schön was indeed guilty of data falsification. Not only was he the first author on the problematic articles, but he alone among his co-authors had access to the experimental devices that produced the disturbingly coincident outcomes. Moreover, it was Schön’s responsibility to keep the experimental protocols for these research papers. Yet the protocols mysteriously vanished when the panel requested them for review. The circumstantial evidence connected with this case not only underwrote a design inference but established Schön as the designer responsible.
And from Physics to Parapsychology
As a final example of where data falsification becomes an issue facing science, consider efforts to debunk parapsychology. Parapsychological experiments attempt to show that parapsychological phenomena are real by producing a specified event of small probability. Persuaded that they’ve produced such an event, parapsychological researchers then explain it in terms of a quasi-design-like theoretical construct called psi (i.e., a non-chance factor or faculty supposedly responsible for such events).
For instance, shuffle some cards and then have a human subject guess their order. Subjects rarely, if ever, guess the correct order with 100 percent accuracy. But to the degree that a subject guesses correctly, the improbability of this coincidence (which will then constitute a specified event of small probability) is regarded as evidence for psi. In attributing such coincidences to psi, the parapsychologist will draw a design inference. The debunker’s task, conversely, will then be to block the parapsychologist’s design inference. In practice, this will mean one of two things: either showing that sloppy experimental method was used that somehow signaled to the subject the order of the cards and thereby enabled the subject, perhaps inadvertently, to overcome chance; or else showing that the experimenter acted fraudulently, whether by making up the data or by otherwise massaging the data to provide evidence for psi. Note that the debunker is as much engaged in drawing a design inference as the parapsychologist — it’s just that one implicates the parapsychologist in fraud, the other implicates psi.
Keeping Science Honest
The takeaway is that science needs the design inference to keep itself honest. In the years since the first edition of this book was published, reports of fraud in science have continued to accumulate. The publish-or-perish mentality that incentivizes inflating the number of one’s publications regardless of quality has only gotten worse. That mentality moves easily from a haste-makes-waste sloppiness to self-serving fudginess to full-orbed fraudulence. Data falsification and other forms of scientific fraud, such as plagiarism, are far too common in science. What keeps scientific fraud in check is our ability to detect it, and it’s the design inference that does the detecting.
We’ve now seen that the design inference makes design readily detectable in everyday life. Moreover, we’ve just seen that its ability to make design detectable in the data of science is central to keeping scientists honest. The grand ambition of this book is to show that the design inference makes design part of the very fabric of science.