Evolution
Just How Well Does That Cherry-Picked Data Fit an Evolutionary Tree?
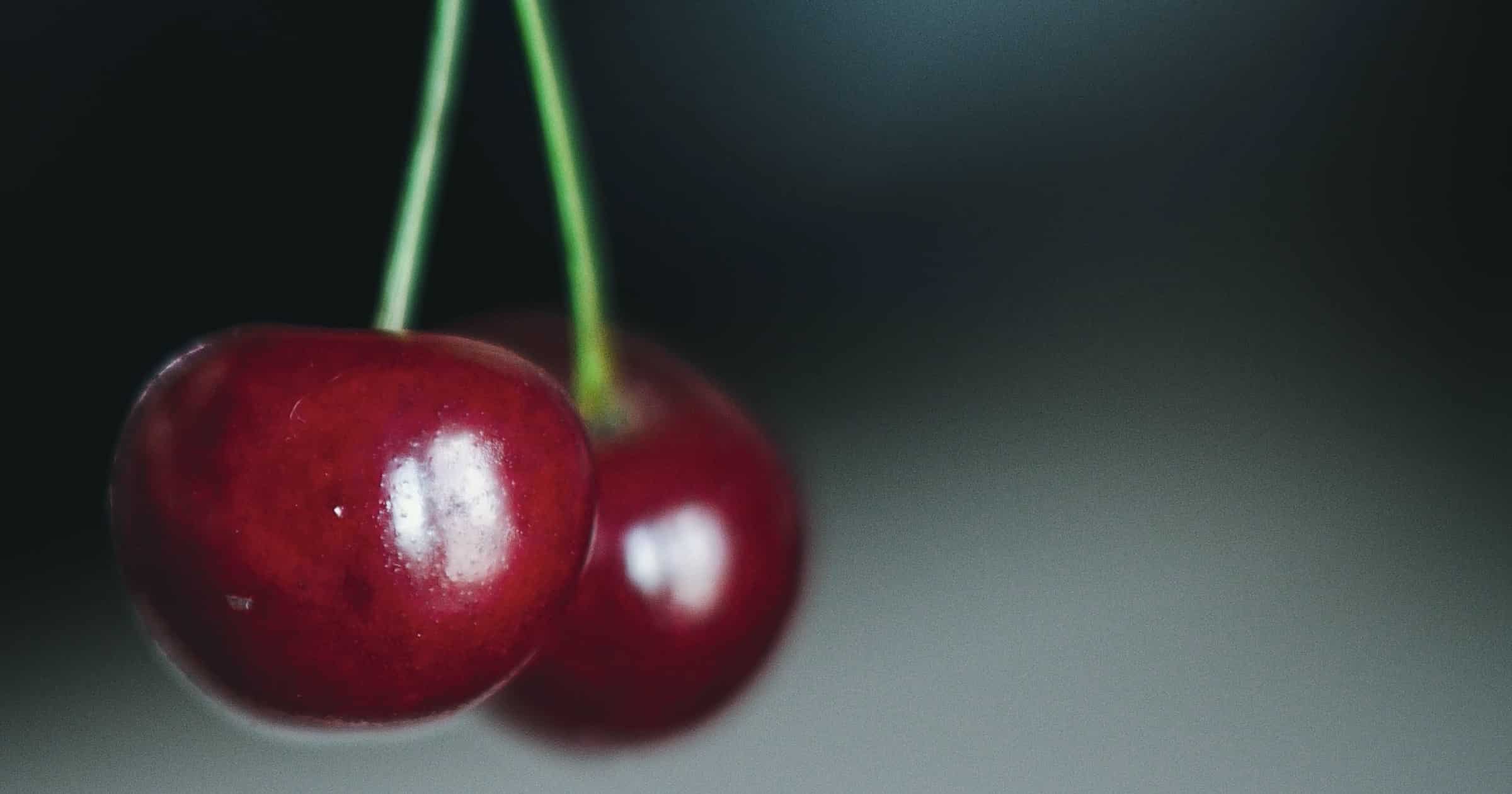
In my previous post, here, responding to YouTuber Gutsick Gibbon, aka Erika, I offered an example with five of the Perelman genes to illustrate how the separate ancestry model was created in the Baum et al. (2016) paper. In the unshuffled example, for clarity, I gave all the synapomorphies perfect consistency across the various genes in different organisms. Said another way, no matter what gene you studied, organism 1 and organism 2 were always the most closely related, followed by organism 3 and finally organism 4. I did this for clarity, but that is not how it occurs in real life. Conflicts between gene-based trees are notoriously common.
An Ideal World
In looking at the Perelman data set, for the 9 of the 54 genes from Murphy et al. (2001), we can see how well all the synapomorphies fit the proposed evolutionary tree by looking at their consistency indexes. Consistency indexes (CI) are calculated by taking the minimum possible number of steps to build the tree, divided by the observed number of steps. In an ideal world, common ancestry would lead you to expect that CI’s would fall close to 1, because the minimum possible number of steps should be close to the observed. In this case, a CI of 1 would mean that all 54 genes fit consistently within the same primate evolutionary tree. A CI of 0 suggests that similarities are no better than completely random data.
If you look at Table 1 from Murphy et al. you will see that the estimated consistency indexes for 16 gene-based trees are between .25 and .65 with the average being around .40. Given that the average CI of these genes (.40) is closer to 0 than to 1, this is not favorable for the common ancestry hypothesis — a CI of 0.4 essentially means that 60 percent of the data did not fit a treelike pattern!
What Does This Mean?
This means that the tree-like pattern from the dataset was not very strong. Of course, if you shuffle it, it looks strong compared to randomness, but it isn’t actually very strong. So we are in a strange “gray area” of the results where the data is more treelike than Gutsick Gibbon thinks a designed dataset should be, but less treelike than an ID proponent like me thinks it should be if it were produced by unguided descent with modification. Who is right? Well, this much I know: Gutsick Gibbon and Baum et al. (2016) have imposed a totally unrealistic constraint upon a designed dataset: namely that traits/synapomorphies must vary at random, without any regard for the fact that correlations between traits are needed to fulfill the requirements of functional systems. These correlations will always give a higher-than-random CI. Meanwhile, the CI’s are so low that on average 60 percent of the data does not fit the treelike pattern predicted by common ancestry. I think it’s clear which model is better explaining these modest CI’s: common design.
I would further bet that pro-ID computer scientist Winston Ewert’s dependency graph (which you can read about here) would provide a much better fit to this data. Speaking of Ewert’s model, in my next post I will deal with Gutsick’s claim that the dependency graph isn’t a real model.