Intelligent Design
Examining Randy Isaac’s Critique of Introduction to Evolutionary Informatics
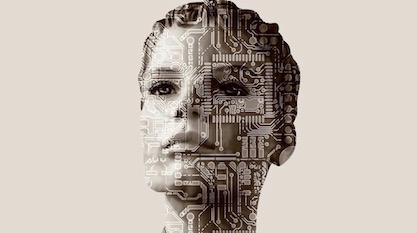
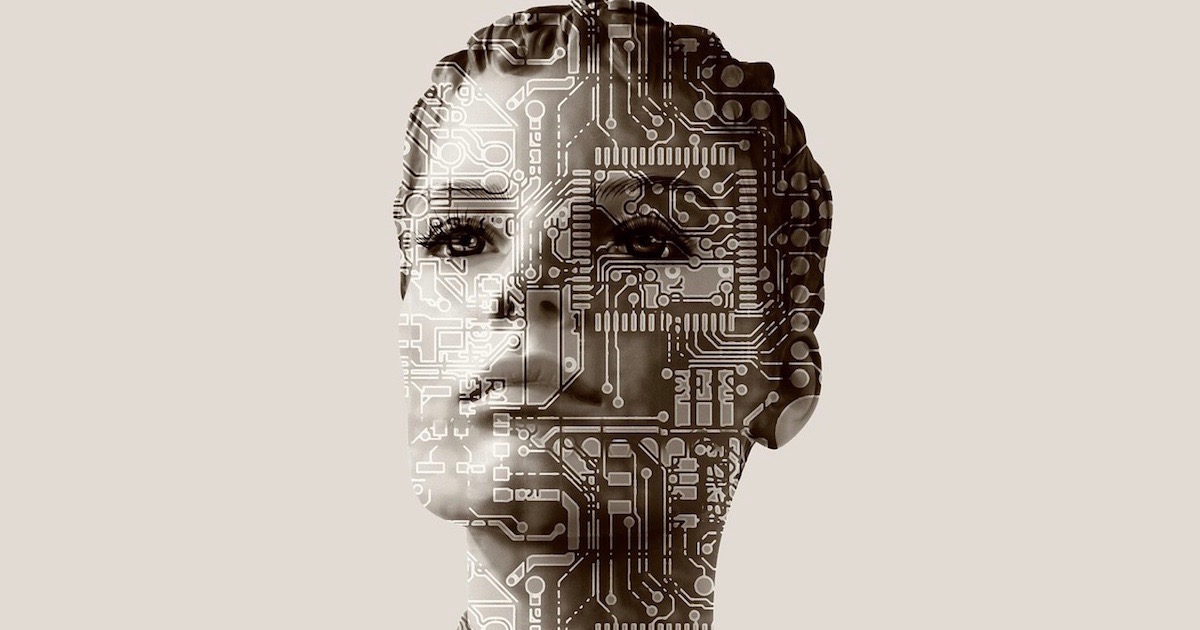
In an article yesterday I examined physicist Randy Isaac’s criticisms of several science chapters in Theistic Evolution: A Scientific, Philosophical, and Theological Critique. Today, I will discuss his critique of the content of the TE book that draws from Introduction to Evolutionary Informatics by Robert Marks, Bill Dembski, and Winston Ewert (MDE). The authors’ work is particularly significant since it demonstrates that no evolutionary process could achieve novel outcomes which correspond to generating significant amounts of new information. Therefore, the standard evolutionary model is incapable of driving major transformations such as a fish evolving into an amphibian.
To fully understand the importance of their research, some historical context is needed. Computer scientists began devising search algorithms modeling biological evolution in an attempt to find new methods to discover solutions to complex problems. These evolutionary algorithms randomly modify details in trial solutions, such as the aerodynamics of a car frame, to solve some problem, such as discovering new car designs with improved fuel efficiency. The algorithms then select the best individual trials based on some fitness function. Those not selected are removed, and the selected ones are duplicated and further modified for the next iteration. The process repeats until a trial meets some predetermined criteria, such as a target fuel efficiency, or solutions stop improving.
Avida and Other Algorithms
Algorithms were eventually designed to demonstrate how evolutionary processes could create novel outcomes. A classic example is the program Avida which could locate in its search space complex functions. Such results have been presented to the public as proof that biological evolution could drive dramatic transformations. However, MDE demonstrated that these programs were only capable of producing complex solutions because the programmers imbedded in the search algorithms information which guide the populations of trials toward desired target outcomes. Without such information, the programs invariably fail to yield anything truly novel.
In addition, MDE drew upon “no free lunch” theorems to demonstrate that no search algorithm could on average find a target any faster than a random search. As a result, evolutionary processes should not be expected to discover complex innovations since the search space is unimaginably large, and natural selection has no access to information related to distant biological outcomes. Relatedly, producing complex innovations corresponds to generating large quantities of new genetic information, and only comparatively miniscule quantities can be obtained through undirected searches. The authors’ work was so convincing that many abandoned using search algorithms as evidence for biological evolution. They instead engaged in historical revisionism by denying that such programs were even relevant to the discussion.
Assumptions and Obfuscations
Isaac should have recognized the authors’ accomplishments, if he had the freedom to honestly evaluate their work. Unfortunately, his philosophical assumptions compelled him to dismiss their results. As a consequence, he engages in obfuscation by arguing that information is physical, so physical processes could generate it for free. He continues by asserting that the genetic code is also physical and not a formal abstraction. And, he raises several issues to unnecessarily complicate the discussion, such as the differences between meaningful and nonmeaningful information, the roles of populations of different species in selection, the exact nature of “goals” in biological evolution, and the potential role of information associated with the state of the environment and local populations. However, none of his concerns challenge MDE’s main conclusions, particularly in the context of the origination of new body plans (design architectures) in the higher taxonomic categories (phyla and classes).
Contrary to Isaac’s assertions, theorists have consistently recognized that the structures in biology which contain information may be physical (e.g., DNA), but the stored information is nonphysical. This distinction has been well articulated by such theorists are Michael Polanyi and Peter Wills. In addition, the nonphysical nature of the genetic code has been widely recognized since the time Crick and Watson first identified the structure of DNA. The code may be implemented through physical mechanisms, but the same holds true for ASCII code in computers and Morse code in the telegraph. Codes are always formal abstractions that can be disassociated from the mediums and mechanisms that implement them.
In addition, the origination of a higher taxonomic group (e.g., trilobites) requires the creation of large numbers of new genes in geological instances. Generating this information involves mutations randomly altering the letters (A, C, D, and T) in different sections of DNA as a population searches for a new set of functional genes with their corresponding proteins. This process sufficiently mimics evolutionary algorithms for MDE’s analyses to fully apply. In particular, the authors developed the algorithmic specified complexity (ASC) measure for quantifying in a pattern meaningful information, and they determined the upper bound for the amount of ASC that could be achieved through any search algorithm. They applied their measure to an individual protein and calculated that the information content of even a minimally functional one exceeds what could ever be produced by any undirected process.
A Pile of Scrabble Pieces
And contrary to Isaac’s suggestion, information related to the state of the environment or to local populations could no more assist in assembling the correct information-rich genetic sequences than the information in the environment of a pile of Scrabble pieces could help arrange the letters into the preamble to the Declaration of Independence. Natural selection resulting from external factors could not guide the search for new genetic information until sufficient numbers of genes became fully functional and activated at the rights times in the right contexts to properly integrate with each other and with existing genetic networks. Only then could some new complex trait, such as an eye lens, improve fitness. Nonfunctional genetic sequences provide no selective advantage, and even functional ones would be useless if they were not fully integrated into the genome.
Moreover, where biological evolution differs from computer algorithms, the challenges do not lessen but amplify. In particular, any evolutionary process directing a major transformation would perform far worse than a purely random search due to the catastrophic consequences of altering the genetic networks that direct body plan formation. The uniform evidence from mutations in developmental genes conclusively demonstrate that distinct body plans represent exceedingly rare targets in a vast sea of nonviable intermediates. Therefore, an evolving population in the evolutionary fitness landscape would always remain stranded on a local peak corresponding to the existing design architecture.
Randy Isaac unjustly devalues the compelling arguments presented by MDE and instead summarizes his critique by stating that “anyone seeking insight into biological or chemical evolution is advised to look elsewhere.”
If he were ever able to break free from his philosophical blinders, he would recognize that the converging evidence from computational, mathematical, and biological disciplines are demonstrating beyond reasonable doubt that evolutionary processes are only able to produce minor refinements of existing traits. And creating anything truly novel requires the direct guidance of a mind.
Image credit: geralt, via Pixabay.